Note
Go to the end to download the full example code.
Catalog-based Forecast Evaluation
This example shows how to evaluate a catalog-based forecasting using the Number test. This test is the simplest of the evaluations.
- Overview:
Define forecast properties (time horizon, spatial region, etc).
Access catalog from ComCat
Filter catalog to be consistent with the forecast properties
Apply catalog-based number test to catalog
Visualize results for catalog-based forecast
Load required libraries
Most of the core functionality can be imported from the top-level csep
package. Utilities are available from the
csep.utils
subpackage.
import csep
from csep.core import regions, catalog_evaluations
from csep.utils import datasets, time_utils
Define start and end times of forecast
Forecasts should define a time horizon in which they are valid. The choice is flexible for catalog-based forecasts, because the catalogs can be filtered to accommodate multiple end-times. Conceptually, these should be separate forecasts.
start_time = time_utils.strptime_to_utc_datetime("1992-06-28 11:57:35.0")
end_time = time_utils.strptime_to_utc_datetime("1992-07-28 11:57:35.0")
Define spatial and magnitude regions
Before we can compute the bin-wise rates we need to define a spatial region and a set of magnitude bin edges. The magnitude
bin edges # are the lower bound (inclusive) except for the last bin, which is treated as extending to infinity. We can
bind these # to the forecast object. This can also be done by passing them as keyword arguments
into csep.load_catalog_forecast()
.
# Magnitude bins properties
min_mw = 4.95
max_mw = 8.95
dmw = 0.1
# Create space and magnitude regions. The forecast is already filtered in space and magnitude
magnitudes = regions.magnitude_bins(min_mw, max_mw, dmw)
region = regions.california_relm_region()
# Bind region information to the forecast (this will be used for binning of the catalogs)
space_magnitude_region = regions.create_space_magnitude_region(region, magnitudes)
Load catalog forecast
To reduce the file size of this example, we’ve already filtered the catalogs to the appropriate magnitudes and spatial locations. The original forecast was computed for 1 year following the start date, so we still need to filter the catalog in time. We can do this by passing a list of filtering arguments to the forecast or updating the class.
By default, the forecast loads catalogs on-demand, so the filters are applied as the catalog loads. On-demand means that until we loop over the forecast in some capacity, none of the catalogs are actually loaded.
More fine-grain control and optimizations can be achieved by creating a csep.core.forecasts.CatalogForecast
directly.
forecast = csep.load_catalog_forecast(datasets.ucerf3_ascii_format_landers_fname,
start_time = start_time, end_time = end_time,
region = space_magnitude_region,
apply_filters = True)
# Assign filters to forecast
forecast.filters = [f'origin_time >= {forecast.start_epoch}', f'origin_time < {forecast.end_epoch}']
Obtain evaluation catalog from ComCat
The csep.core.forecasts.CatalogForecast
provides a method to compute the expected number of events in spatial cells. This
requires a region with magnitude information.
We need to filter the ComCat catalog to be consistent with the forecast. This can be done either through the ComCat API or using catalog filtering strings. Here we’ll use the ComCat API to make the data access quicker for this example. We still need to filter the observed catalog in space though.
# Obtain Comcat catalog and filter to region.
comcat_catalog = csep.query_comcat(start_time, end_time, min_magnitude=forecast.min_magnitude)
# Filter observed catalog using the same region as the forecast
comcat_catalog = comcat_catalog.filter_spatial(forecast.region)
print(comcat_catalog)
# Plot the catalog
comcat_catalog.plot()
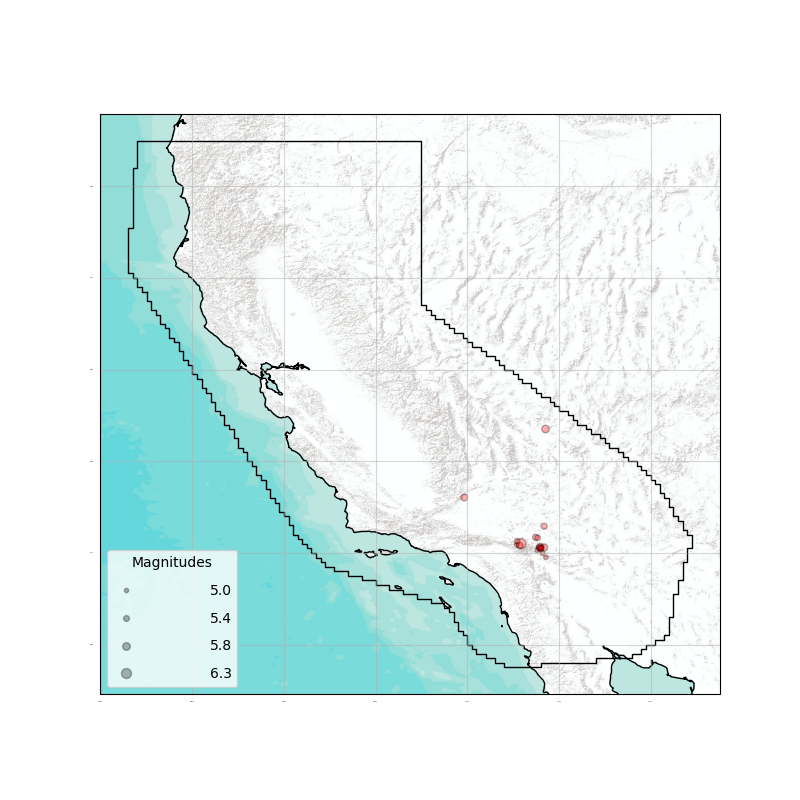
Fetched ComCat catalog in 0.20955705642700195 seconds.
Downloaded catalog from ComCat with following parameters
Start Date: 1992-06-28 12:00:45+00:00
End Date: 1992-07-24 18:14:36.250000+00:00
Min Latitude: 33.901 and Max Latitude: 36.705
Min Longitude: -118.067 and Max Longitude: -116.285
Min Magnitude: 4.95
Found 19 events in the ComCat catalog.
Name: None
Start Date: 1992-06-28 12:00:45+00:00
End Date: 1992-07-24 18:14:36.250000+00:00
Latitude: (33.901, 36.705)
Longitude: (-118.067, -116.285)
Min Mw: 4.95
Max Mw: 6.3
Event Count: 19
<GeoAxes: >
Perform number test
We can perform the Number test on the catalog based forecast using the observed catalog we obtained from Comcat.
number_test_result = catalog_evaluations.number_test(forecast, comcat_catalog)
Processed 1 catalogs in 0.0012786388397216797 seconds
Processed 2 catalogs in 0.0017483234405517578 seconds
Processed 3 catalogs in 0.002138376235961914 seconds
Processed 4 catalogs in 0.0024394989013671875 seconds
Processed 5 catalogs in 0.0026862621307373047 seconds
Processed 6 catalogs in 0.00304412841796875 seconds
Processed 7 catalogs in 0.0033330917358398438 seconds
Processed 8 catalogs in 0.0037136077880859375 seconds
Processed 9 catalogs in 0.004663944244384766 seconds
Processed 10 catalogs in 0.005013942718505859 seconds
Processed 20 catalogs in 0.008730888366699219 seconds
Processed 30 catalogs in 0.012635946273803711 seconds
Processed 40 catalogs in 0.01632857322692871 seconds
Processed 50 catalogs in 0.020423173904418945 seconds
Processed 60 catalogs in 0.02383279800415039 seconds
Processed 70 catalogs in 0.02713775634765625 seconds
Processed 80 catalogs in 0.03069162368774414 seconds
Processed 90 catalogs in 0.0344538688659668 seconds
Processed 100 catalogs in 0.03769087791442871 seconds
Processed 200 catalogs in 0.06992959976196289 seconds
Processed 300 catalogs in 0.10522913932800293 seconds
Processed 400 catalogs in 0.1394977569580078 seconds
Processed 500 catalogs in 0.2021493911743164 seconds
Processed 600 catalogs in 0.235670804977417 seconds
Processed 700 catalogs in 0.26974058151245117 seconds
Processed 800 catalogs in 0.3357207775115967 seconds
Processed 900 catalogs in 0.36815333366394043 seconds
Processed 1000 catalogs in 0.4017794132232666 seconds
Processed 2000 catalogs in 0.8729867935180664 seconds
Processed 3000 catalogs in 1.298497200012207 seconds
Processed 4000 catalogs in 1.7711167335510254 seconds
Processed 5000 catalogs in 2.2091479301452637 seconds
Processed 6000 catalogs in 2.6439967155456543 seconds
Processed 7000 catalogs in 3.1056087017059326 seconds
Processed 8000 catalogs in 3.516176462173462 seconds
Processed 9000 catalogs in 4.006390571594238 seconds
Processed 10000 catalogs in 4.435344457626343 seconds
Plot number test result
We can create a simple visualization of the number test from the evaluation result class.
ax = number_test_result.plot(show=True)
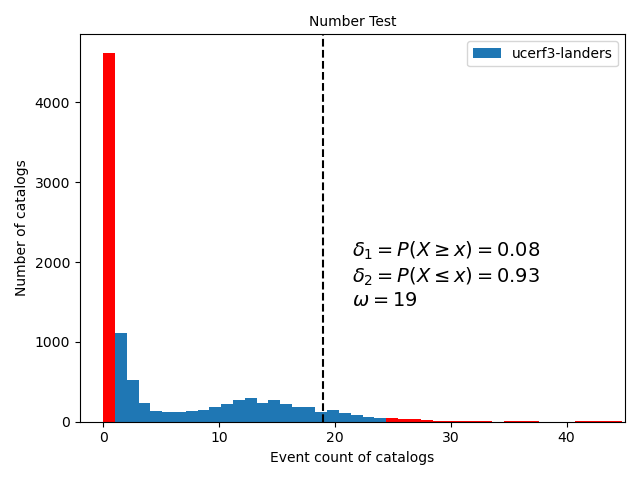
Total running time of the script: (0 minutes 8.946 seconds)