Note
Go to the end to download the full example code
Plot customizations
This example shows how to include some advanced options in the spatial visualization of Gridded Forecasts and Evaluation Results
- Overview:
Define optional plotting arguments
Set extent of maps
Visualizing selected magnitude bins
Plot global maps
Plot multiple Evaluation Results
Example 1: Spatial dataset plot arguments
Load required libraries
import csep
import cartopy
import numpy
from csep.utils import datasets, plots
import matplotlib.pyplot as plt
Load a Grid Forecast from the datasets
forecast = csep.load_gridded_forecast(datasets.hires_ssm_italy_fname,
name='Werner, et al (2010) Italy')
Selecting plotting arguments
Create a dictionary containing the plot arguments
args_dict = {'title': 'Italy 10 year forecast',
'grid_labels': True,
'borders': True,
'feature_lw': 0.5,
'basemap': 'ESRI_imagery',
'cmap': 'rainbow',
'alpha_exp': 0.8,
'projection': cartopy.crs.Mercator()}
These arguments are, in order:
Assign a title
Set labels to the geographic axes
Draw country borders
Set a linewidth of 0.5 to country borders
Select ESRI Imagery as a basemap.
Assign
'rainbow'
as colormap. Possible values from frommatplotlib.cm
libraryDefines 0.8 for an exponential transparency function (default is 0 for constant alpha, whereas 1 for linear).
An object cartopy.crs.Projection() is passed as Projection to the map
The complete description of plot arguments can be found in csep.utils.plots.plot_spatial_dataset()
Plotting the dataset
The map extent can be defined. Otherwise, the extent of the data would be used. The dictionary defined must be passed as argument
ax = forecast.plot(extent=[3, 22, 35, 48],
show=True,
plot_args=args_dict)
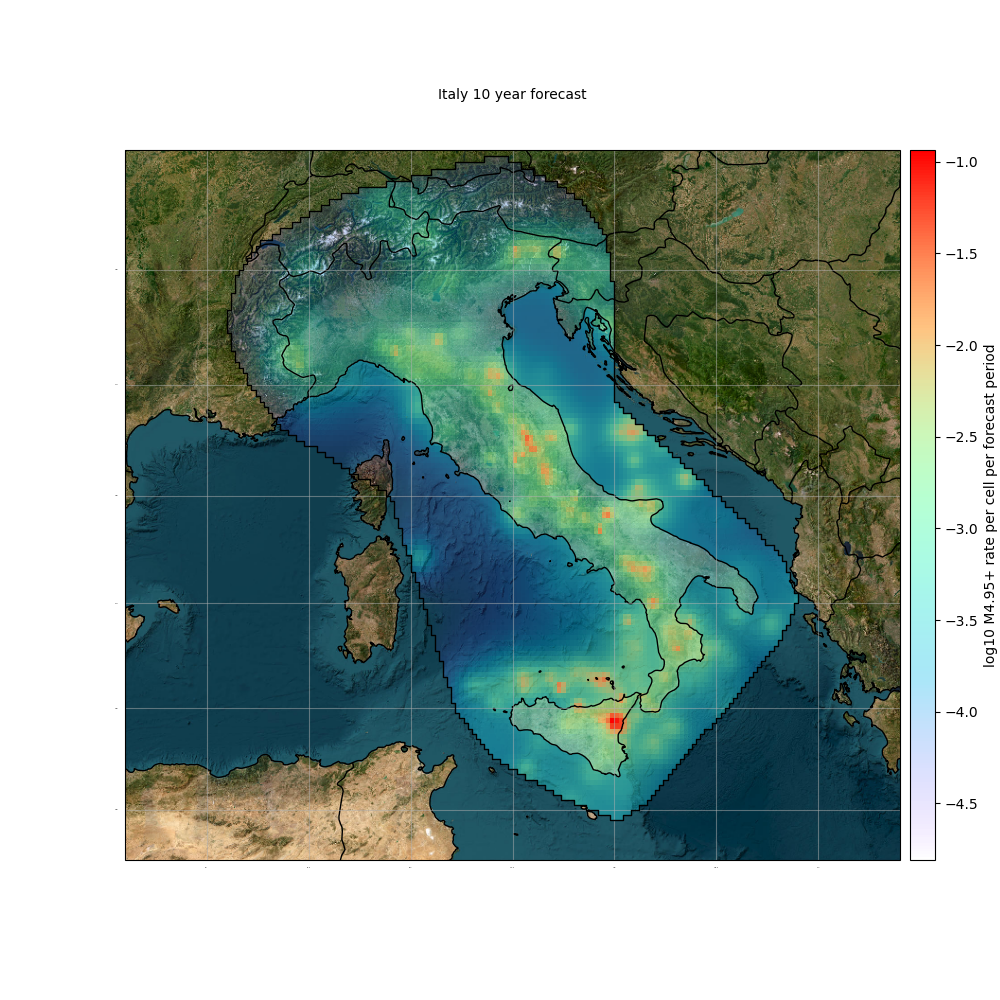
Example 2: Plot a global forecast and a selected magnitude bin range
Load a Global Forecast from the datasets
A downsampled version of the GEAR1 forecast can be found in datasets.
forecast = csep.load_gridded_forecast(datasets.gear1_downsampled_fname,
name='GEAR1 Forecast (downsampled)')
Filter by magnitudes
We get the rate of events of 5.95<=M_w<=7.5
low_bound = 6.15
upper_bound = 7.55
mw_bins = forecast.get_magnitudes()
mw_ind = numpy.where(numpy.logical_and( mw_bins >= low_bound, mw_bins <= upper_bound))[0]
rates_mw = forecast.data[:, mw_ind]
We get the total rate between these magnitudes
The data is stored in a 1D array, so it should be projected into region 2D cartesian grid.
rate_sum = forecast.region.get_cartesian(rate_sum)
Define plot arguments
We define the arguments and a global projection, centered at $lon=-180$
plot_args = {'figsize': (10,6), 'coastline':True, 'feature_color':'black',
'projection': cartopy.crs.Robinson(central_longitude=180.0),
'title': forecast.name, 'grid_labels': False,
'cmap': 'magma',
'clabel': r'$\log_{10}\lambda\left(M_w \in [{%.2f},\,{%.2f}]\right)$ per '
r'${%.1f}^\circ\times {%.1f}^\circ $ per forecast period' %
(low_bound, upper_bound, forecast.region.dh, forecast.region.dh)}
Plotting the dataset
To plot a global forecast, we must assign the option set_global=True
, which is required by :ref:cartopy to handle
internally the extent of the plot
ax = plots.plot_spatial_dataset(numpy.log10(rate_sum), forecast.region,
show=True, set_global=True,
plot_args=plot_args)
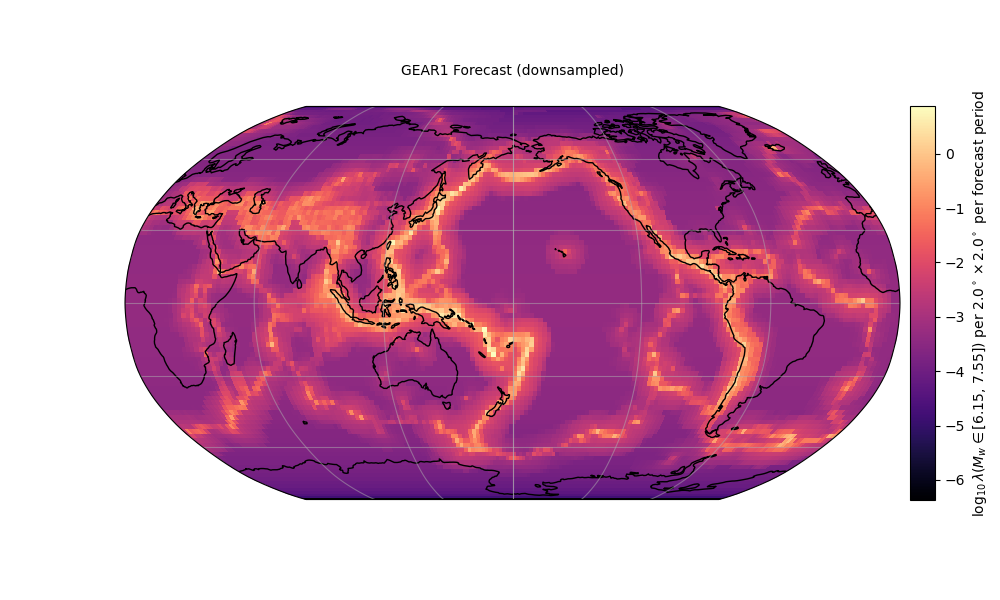
Example 3: Plot a catalog
Load a Catalog from ComCat
start_time = csep.utils.time_utils.strptime_to_utc_datetime('1995-01-01 00:00:00.0')
end_time = csep.utils.time_utils.strptime_to_utc_datetime('2015-01-01 00:00:00.0')
min_mag = 3.95
catalog = csep.query_comcat(start_time, end_time, min_magnitude=min_mag, verbose=False)
# **Define plotting arguments**
plot_args = {'basemap': 'ESRI_terrain',
'markersize': 2,
'markercolor': 'red',
'alpha': 0.3,
'mag_scale': 7,
'legend': True,
'legend_loc': 3,
'mag_ticks': [4.0, 5.0, 6.0, 7.0]}
Fetched ComCat catalog in 22.360023736953735 seconds.
These arguments are, in order:
Assign as basemap the ESRI_terrain webservice
Set minimum markersize of 2 with red color
Set a 0.3 transparency
mag_scale is used to exponentially scale the size with respect to magnitude. Recommended 1-8
Set legend True and location in 3 (lower-left corner)
Set a list of Magnitude ticks to display in the legend
The complete description of plot arguments can be found in csep.utils.plots.plot_catalog()
# **Plot the catalog**
ax = catalog.plot(show=False, plot_args=plot_args)
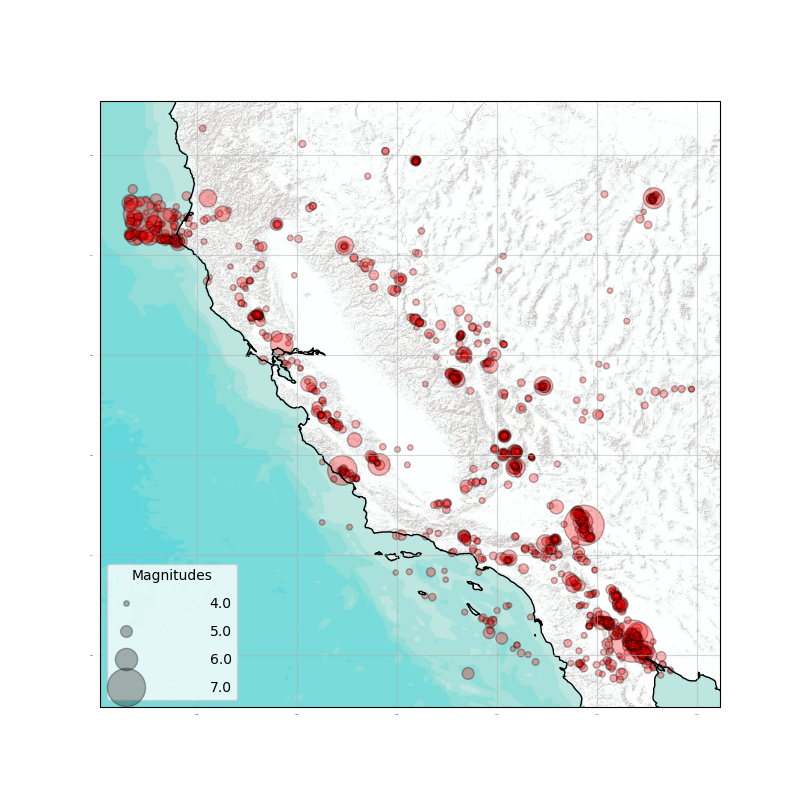
Example 4: Plot multiple evaluation results
Load L-test results from example .json files (See Grid-based Forecast Evaluation for information on calculating and storing evaluation results)
L_results = [csep.load_evaluation_result(i) for i in datasets.l_test_examples]
args = {'figsize': (6,5),
'title': r'$\mathcal{L}-\mathrm{test}$',
'title_fontsize': 18,
'xlabel': 'Log-likelihood',
'xticks_fontsize': 9,
'ylabel_fontsize': 9,
'linewidth': 0.8,
'capsize': 3,
'hbars':True,
'tight_layout': True}
Description of plot arguments can be found in plot_poisson_consistency_test()
.
We set one_sided_lower=True
as usual for an L-test, where the model is rejected if the observed
is located within the lower tail of the simulated distribution.
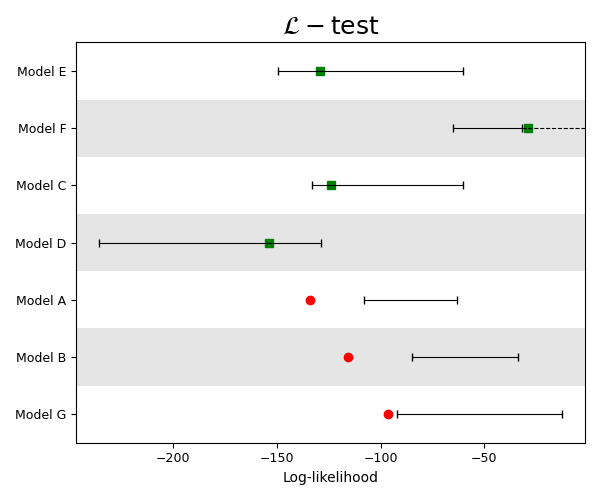
Total running time of the script: (0 minutes 49.988 seconds)