Note
Go to the end to download the full example code.
Working with catalog-based forecasts
This example shows some basic interactions with data-based forecasts. We will load in a forecast stored in the CSEP data format, and compute the expected rates on a 0.1° x 0.1° grid covering the state of California. We will plot the expected rates in the spatial cells.
- Overview:
Define forecast properties (time horizon, spatial region, etc).
Compute the expected rates in space and magnitude bins
Plot expected rates in the spatial cells
Load required libraries
Most of the core functionality can be imported from the top-level csep
package. Utilities are available from the
csep.utils
subpackage.
import numpy
import csep
from csep.core import regions
from csep.utils import datasets
Load data forecast
PyCSEP contains some basic forecasts that can be used to test of the functionality of the package. This forecast has already been filtered to the California RELM region.
Define spatial and magnitude regions
Before we can compute the bin-wise rates we need to define a spatial region and a set of magnitude bin edges. The magnitude
bin edges # are the lower bound (inclusive) except for the last bin, which is treated as extending to infinity. We can
bind these # to the forecast object. This can also be done by passing them as keyword arguments
into csep.load_catalog_forecast()
.
# Magnitude bins properties
min_mw = 4.95
max_mw = 8.95
dmw = 0.1
# Create space and magnitude regions
magnitudes = regions.magnitude_bins(min_mw, max_mw, dmw)
region = regions.california_relm_region()
# Bind region information to the forecast (this will be used for binning of the catalogs)
forecast.region = regions.create_space_magnitude_region(region, magnitudes)
Compute spatial event counts
The csep.core.forecasts.CatalogForecast
provides a method to compute the expected number of events in spatial cells. This
requires a region with magnitude information.
_ = forecast.get_expected_rates(verbose=True)
Processed 1 catalogs in 0.001 seconds
Processed 2 catalogs in 0.002 seconds
Processed 3 catalogs in 0.003 seconds
Processed 4 catalogs in 0.003 seconds
Processed 5 catalogs in 0.004 seconds
Processed 6 catalogs in 0.004 seconds
Processed 7 catalogs in 0.005 seconds
Processed 8 catalogs in 0.005 seconds
Processed 9 catalogs in 0.007 seconds
Processed 10 catalogs in 0.007 seconds
Processed 20 catalogs in 0.013 seconds
Processed 30 catalogs in 0.019 seconds
Processed 40 catalogs in 0.026 seconds
Processed 50 catalogs in 0.032 seconds
Processed 60 catalogs in 0.038 seconds
Processed 70 catalogs in 0.044 seconds
Processed 80 catalogs in 0.051 seconds
Processed 90 catalogs in 0.057 seconds
Processed 100 catalogs in 0.063 seconds
Processed 200 catalogs in 0.120 seconds
Processed 300 catalogs in 0.180 seconds
Processed 400 catalogs in 0.240 seconds
Processed 500 catalogs in 0.330 seconds
Processed 600 catalogs in 0.386 seconds
Processed 700 catalogs in 0.445 seconds
Processed 800 catalogs in 0.537 seconds
Processed 900 catalogs in 0.594 seconds
Processed 1000 catalogs in 0.653 seconds
Processed 2000 catalogs in 1.374 seconds
Processed 3000 catalogs in 2.044 seconds
Processed 4000 catalogs in 2.731 seconds
Processed 5000 catalogs in 3.409 seconds
Processed 6000 catalogs in 4.083 seconds
Processed 7000 catalogs in 4.789 seconds
Processed 8000 catalogs in 5.429 seconds
Processed 9000 catalogs in 6.156 seconds
Processed 10000 catalogs in 6.834 seconds
Plot expected event counts
We can plot the expected event counts the same way that we plot a csep.core.forecasts.GriddedForecast
ax = forecast.expected_rates.plot(plot_args={'clim': [-3.5, 0]}, show=True)
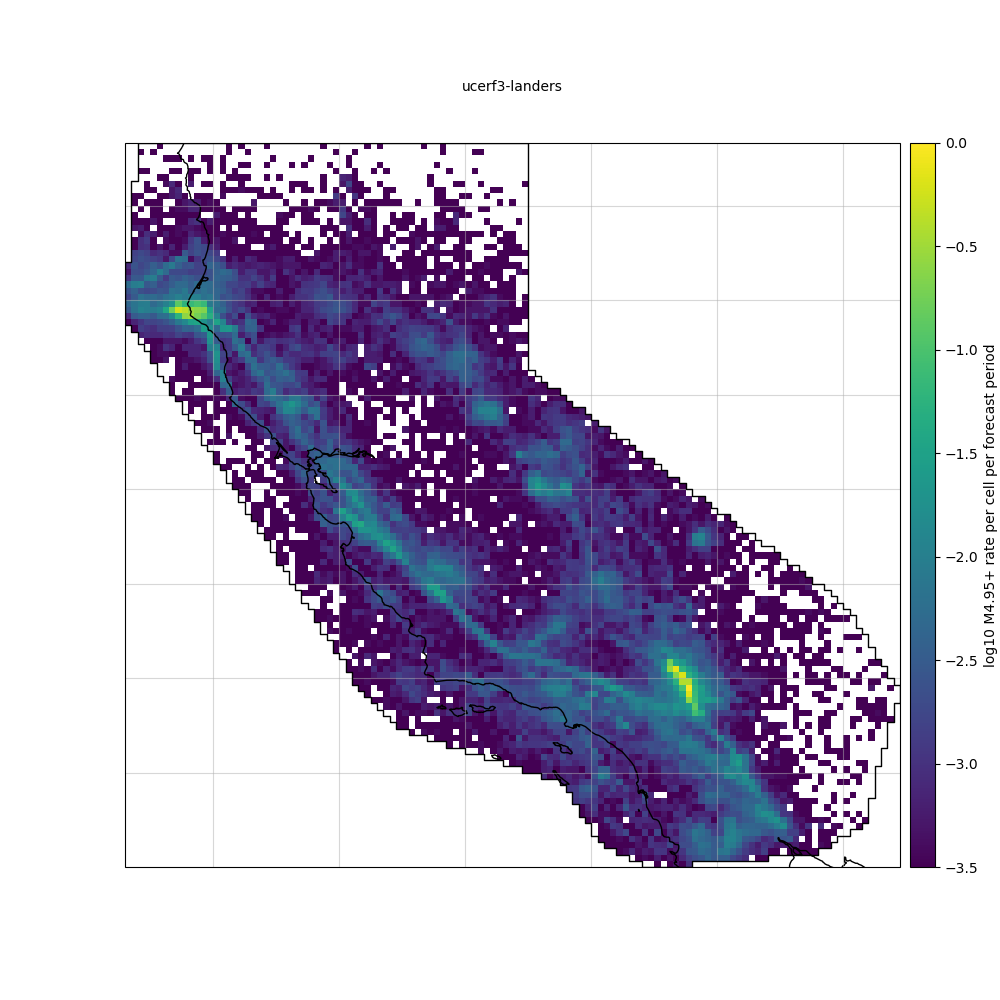
The images holes in the image are due to under-sampling from the forecast.
Quick sanity check
The forecasts were filtered to the spatial region so all events should be binned. We loop through each data in the forecast and count the number of events and compare that with the expected rates. The expected rate is an average in each space-magnitude bin, so we have to multiply this value by the number of catalogs in the forecast.
Total running time of the script: (0 minutes 8.689 seconds)